Hello,
I need to train an ML-model with image segmentation to run on a microcontroller.
For this purpose I chose the path:
- Train a model on Azure ML with my dataset which I labeled
- Convert the trained model to ONNX format
- Convert ONNX model to TensorFlow format (TF)
- Convert TensorFlow model to TensorFlowLight format
The model generated by Azure ML produces really great results with inference, which I can download.
I believe, the model is in PyTorch format, wrapped into MLFlow wrapper.
I was able to transform the model into ONNX format on my local machine. However, when I try to transform ONNX to TF format, I'm getting a lot of different issues. Not sure if the tools I use can't convert, or configuration of the tools, or I generated bad ONNX model.
So, I found that AzureML can also convert trained model into ONNX format, and found this notebook:
https://github.com/Azure/azureml-examples/blob/v1-archive/v1/python-sdk/tutorials/automl-with-azureml/classification-bank-marketing-all-features/auto-ml-classification-bank-marketing-all-features.ipynb
I was trying to follow the steps from the notebook, and selected my previous run with trained model, however got the error:
"Requested an ONNX compatible model but the run has ONNX compatibility disabled."
After that I tried to start a new automated ML job with "Task settings >View additional conf. settings > Onnx normalization" enabled. However this new trained model also has disabled ONNX compatibility.
Then I opened training Experiment, found the best training job (which is the last), tab "Output + Logs", and found thes files: outputs/generated_code/{script_run_notebook.ipynb, script.py}.
In "script.py" I found these properties, and updated all from False to True: "'enable_onnx_compatible_models':True,'enable_onnx_normalization':True,'enable_split_onnx_featurizer_estimator_models':True".
After that I configured and launched script_run_notebook.ipynb notebook.
The notebook seemed to succeed, however, when I open the ML job, I don't see 3 hierarchical jobs like I see if run the job from Azure ML portal. I see just one job and I see the following error in user_logs/std_log.txt:
Type: Unclassified
Class: UnboundLocalError
Message: [Hidden as it may contain PII]
Traceback: File "utilities.py", line 172, in generate_model_code_and_notebook
code = code_generator.generate_code(current_run, pipeline)
File "utilities.py", line 114, in generate_code
return code_generator.generate_verticals_script()
File "code_generator.py", line 161, in generate_verticals_script
filepath = os.path.abspath(filename)
ExceptionTarget: Unspecified
Code generation failed; skipping.
The script duration was 479.75 seconds.
Exception in thread Thread-536:
Traceback (most recent call last):
File "/azureml-envs/azureml-automl-dnn-vision-gpu/lib/python3.9/threading.py", line 973, in _bootstrap_inner
self.run()
File "/azureml-envs/azureml-automl-dnn-vision-gpu/lib/python3.9/threading.py", line 1286, in run
self.function(*self.args, **self.kwargs)
File "/azureml-envs/azureml-automl-dnn-vision-gpu/lib/python3.9/site-packages/azureml/_common/async_utils/daemon.py", line 27, in _do_work
self.work()
File "/azureml-envs/azureml-automl-dnn-vision-gpu/lib/python3.9/site-packages/azureml/_common/async_utils/batch_task_queue.py", line 81, in _do_work
task_queue.add(self._handle_batch)
File "/azureml-envs/azureml-automl-dnn-vision-gpu/lib/python3.9/site-packages/azureml/_common/async_utils/task_queue.py", line 63, in add
future = self.create_future(func, *args, **kwargs)
File "/azureml-envs/azureml-automl-dnn-vision-gpu/lib/python3.9/site-packages/azureml/_common/async_utils/task_queue.py", line 83, in create_future
return self._worker_pool.submit(func, *args, **kwargs)
File "/azureml-envs/azureml-automl-dnn-vision-gpu/lib/python3.9/site-packages/azureml/_common/async_utils/worker_pool.py", line 25, in submit
return super(WorkerPool, self).submit(func, *args, **kwargs)
File "/azureml-envs/azureml-automl-dnn-vision-gpu/lib/python3.9/concurrent/futures/thread.py", line 163, in submit
raise RuntimeError('cannot schedule new futures after '
RuntimeError: cannot schedule new futures after interpreter shutdown
My questions are:
- Is it possible to enable ONNX compatibility in Azure ML portal, or this option only available from SDK?
- I actually see model.onnx file among other intermediate models in training artifacts. Is this the same ONNX model which I can retrieve if ONNX compatibility mode is enabled or is it different?
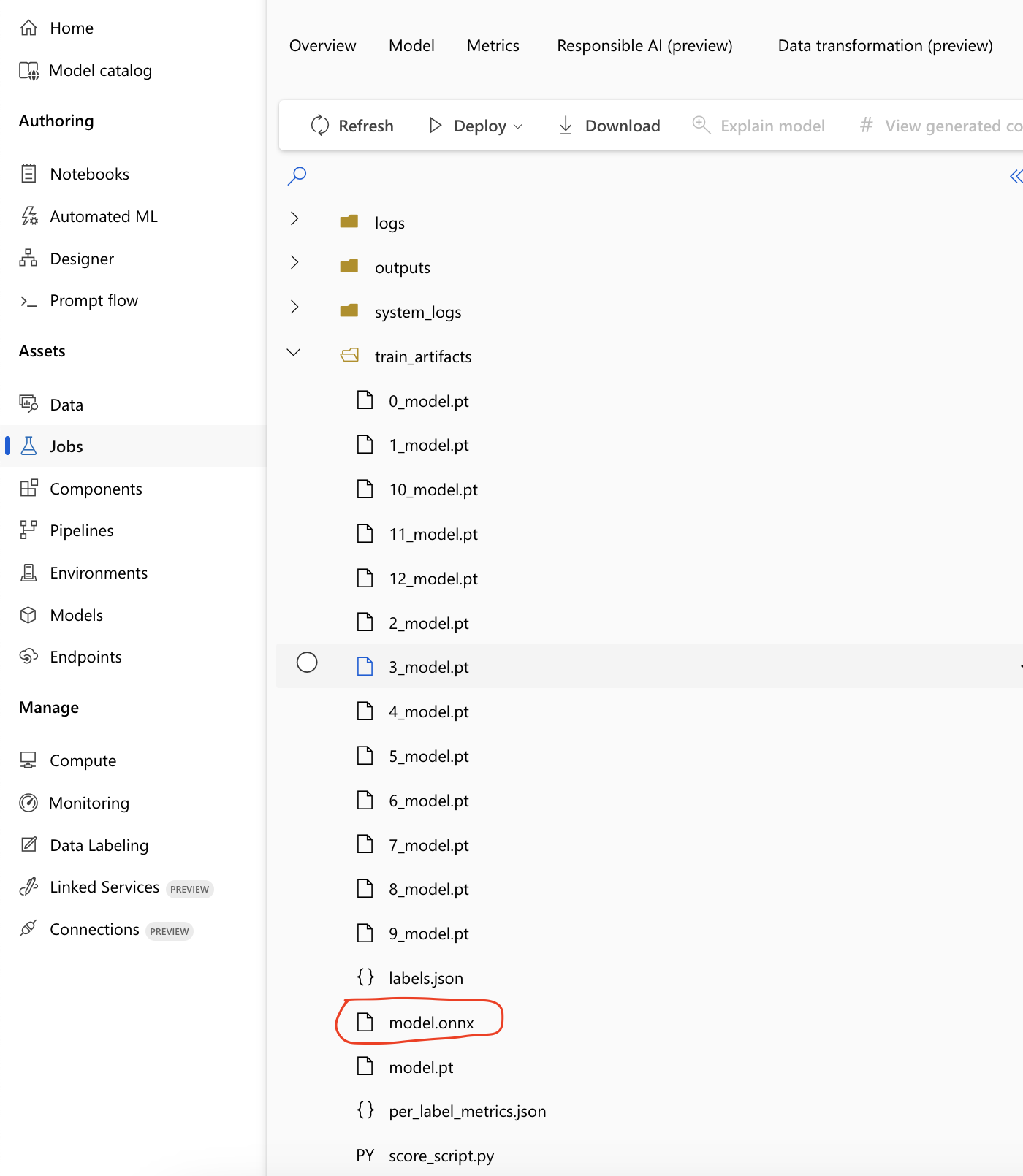
- How can I fix the error I see when I try to run the training job from my notebook?
The notebook is generated by AzureML by clicking "View generated code"
Thank you!